Unlocking the Power of Healthcare Datasets for Machine Learning
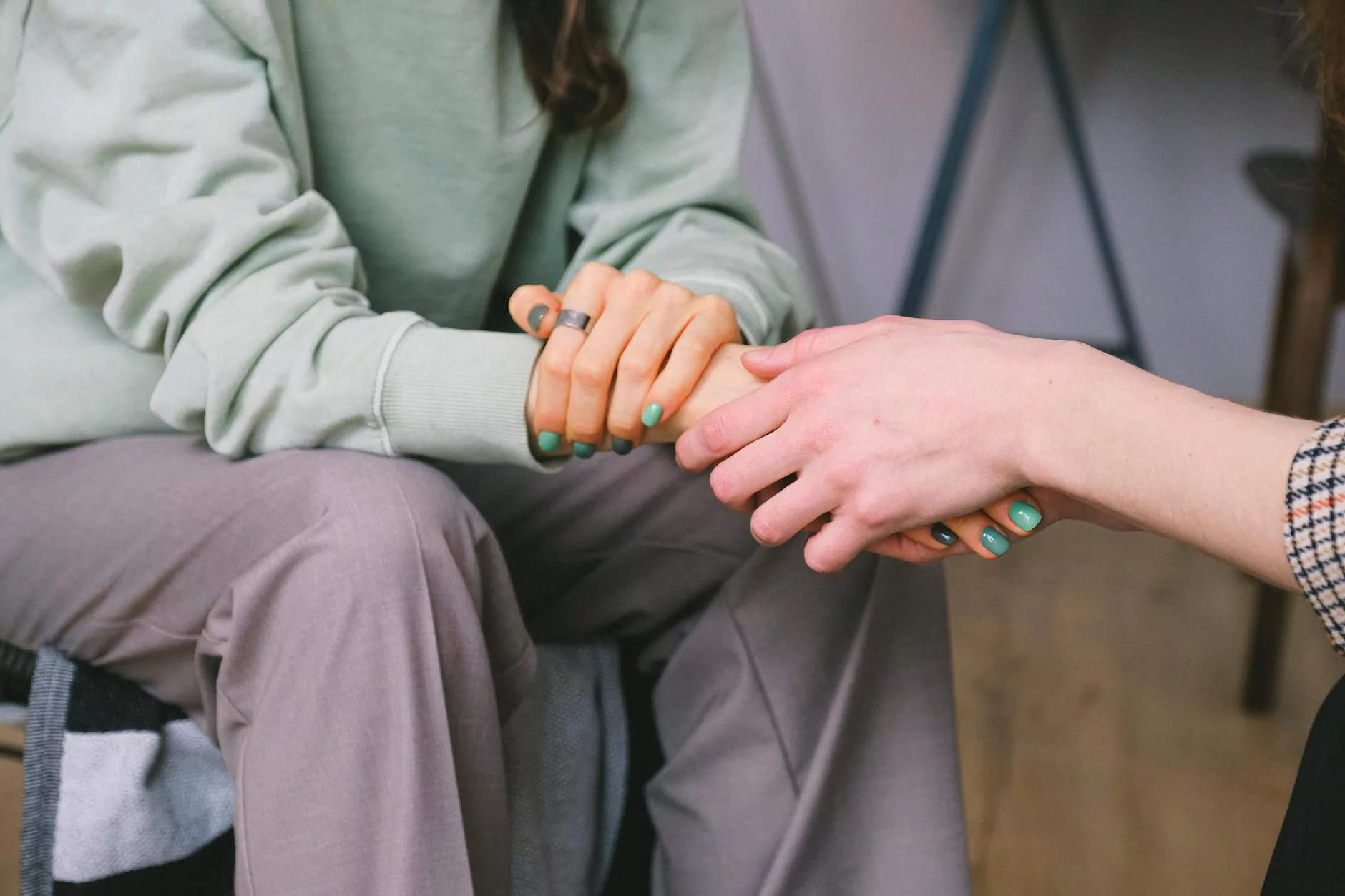
In the era of big data, businesses are increasingly turning to machine learning (ML) to harness vast amounts of information for strategic advantage. One area that shows immense promise is the use of healthcare datasets for machine learning. These datasets provide insights that not only improve patient care but also streamline operations, enhance decision-making, and foster innovation.
The Importance of Healthcare Datasets
Healthcare datasets are rich repositories of structured and unstructured data collected from various sources, including hospitals, clinics, and research studies. Leveraging these datasets can lead to groundbreaking advancements in healthcare delivery. Here are some key points outlining their significance:
- Improved Patient Outcomes: By analyzing patient data, healthcare providers can offer personalized treatments and better manage chronic conditions.
- Operational Efficiency: Machine learning can help identify inefficiencies in healthcare processes, allowing organizations to optimize resource allocation.
- Predictive Analytics: Predictive models can forecast patient admissions and emergency room visits, enabling better planning and staffing.
- Research and Development: Leveraging datasets for ML accelerates the development of new drugs and therapies.
Types of Healthcare Datasets
When considering healthcare datasets for machine learning, it’s important to understand the different types of data available:
1. Electronic Health Records (EHRs)
EHRs contain comprehensive patient histories, medications, allergies, lab results, and treatment plans. They are fundamental to predictive modeling in healthcare.
2. Claims Data
This data includes billing information from insurance companies and provides insights into treatment patterns, costs, and patient demographics.
3. Genomic Data
Genomic datasets allow for the analysis of genetic information, crucial for personalized medicine and identifying population health trends.
4. Wearable Device Data
Data from wearable devices offers real-time health metrics, facilitating continuous monitoring of patients and contributing valuable data for ML algorithms.
5. Public Health Data
Aggregated data collected by government and health organizations provides a broader view of health trends, outbreaks, and the effectiveness of public health initiatives.
Machine Learning Techniques in Healthcare
The effective use of healthcare datasets for machine learning hinges on various ML techniques that can be applied to derive meaningful insights:
1. Supervised Learning
Supervised learning algorithms are trained on labeled data, making them ideal for classification tasks such as diagnosing diseases based on symptoms and lab results.
2. Unsupervised Learning
These algorithms are useful for discovering patterns within data without prior labels. They can identify patient clusters with similar health profiles, enabling targeted interventions.
3. Reinforcement Learning
This technique helps in optimizing treatment protocols by learning from the outcomes of past decisions, continuously improving the decision-making process.
Challenges in Using Healthcare Datasets
Despite the transformative potential of healthcare datasets for machine learning, several challenges persist:
1. Data Privacy and Security
Patient data is highly sensitive, requiring robust measures to protect confidentiality and comply with regulations like HIPAA.
2. Data Quality
Inaccurate, incomplete, or poorly structured data can lead to misleading insights. Ensuring data integrity is crucial for the success of ML applications.
3. Integration of Diverse Data Sources
Bringing together data from various sources, including EHRs and wearable technologies, poses technical challenges, especially in terms of harmonizing formats.
Best Practices for Leveraging Healthcare Datasets
Organizations looking to harness healthcare datasets for machine learning should adhere to several best practices:
- Ensure Data Compliance: Always abide by legal standards and ethical guidelines to protect patient information.
- Focus on Data Quality: Implement thorough data cleaning and validation processes.
- Invest in Data Infrastructure: Build a robust data architecture that supports efficient data storage, retrieval, and processing.
- Engage Interdisciplinary Teams: Collaborate with data scientists, healthcare professionals, and domain experts to derive actionable insights.
- Continuously Monitor Models: Regularly evaluate and update ML models to ensure they remain relevant and accurate over time.
Future Trends in Healthcare Datasets and Machine Learning
The intersection of healthcare and machine learning is rapidly evolving. Here are some emerging trends that will shape the future:
1. Enhanced Personalization
As datasets expand, machine learning models will become more adept at delivering highly personalized patient care, tailored to individual needs and preferences.
2. Real-Time Analytics
The rise of IoT devices in healthcare will facilitate real-time data collection and analysis, providing immediate insights that enhance patient care and operational efficiency.
3. Interoperability Standards
Advances in technology will promote better interoperability between systems, allowing for seamless data sharing and integration across the healthcare ecosystem.
4. Focus on Social Determinants of Health
Machine learning will increasingly incorporate social determinants of health data to provide a more holistic view of patient populations and improve health equity.
Conclusion
In conclusion, the transformative potential of healthcare datasets for machine learning is immense. By effectively leveraging these datasets, organizations can not only improve patient care but also drive operational efficiency and foster innovation. It is crucial for businesses, especially those in the field of software development like keymakr.com, to stay ahead of the curve by investing in robust data practices and advanced machine learning techniques. As the healthcare landscape continues to evolve, those who embrace the power of data will undoubtedly lead the charge toward a healthier future for all.