Transforming Business Operations with **Spam Filtering Using Machine Learning**
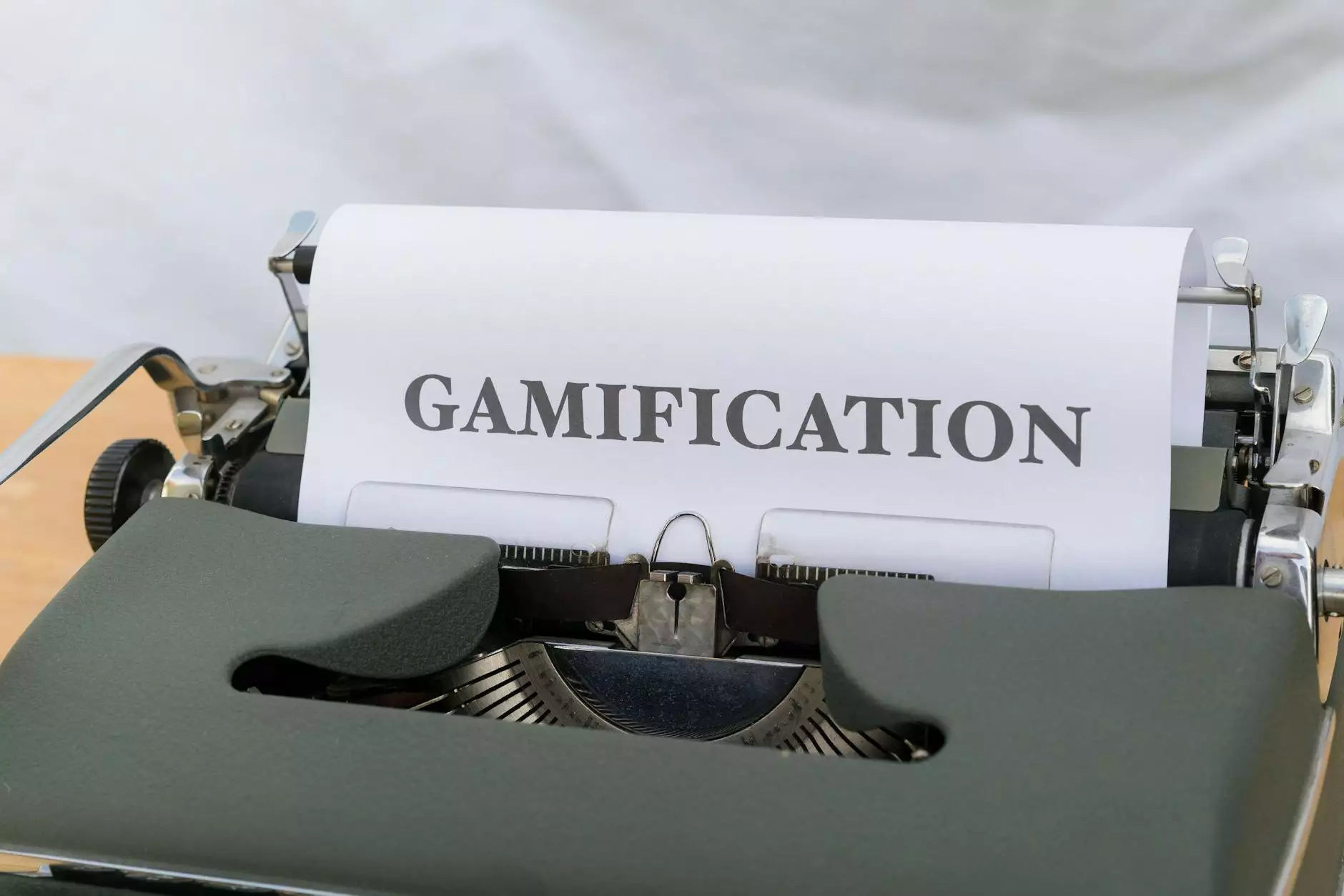
In today's digital world, where the volume of emails and online communications is constantly increasing, businesses face an ever-growing challenge: managing spam effectively. Spam filtering using machine learning has emerged as a powerful solution to this problem, ensuring that organizations can protect their data, enhance their productivity, and maintain a seamless communication experience. This article delves deeply into how this innovative technology works, its benefits, and how it can revolutionize IT services and security systems for businesses.
Understanding the Need for Protection
The challenge of managing spam is not just about having a cluttered inbox. The consequences of unchecked spam can be severe, leading to:
- Data Breaches: Many spam messages contain phishing attempts that can compromise sensitive business information.
- Productivity Loss: Employees can waste valuable time sorting through irrelevant emails.
- Resource Drain: IT teams may spend excessive time managing spam instead of focusing on strategic initiatives.
To combat these challenges, spam filtering has become essential for businesses aiming to streamline their operations and maintain robust security measures.
The Mechanics of Spam Filtering
Spam filtering using machine learning utilizes sophisticated algorithms that analyze incoming emails in real-time. Here are the primary components of this process:
1. Data Collection
Machine learning algorithms require extensive data to learn and improve. Businesses can enhance their spam filters by aggregating email data from various sources, including:
- Incoming emails
- Blocked emails
- User feedback on false positives and negatives
2. Feature Extraction
The next step involves identifying the features that distinguish spam from legitimate emails. These may include:
- Email sender's reputation
- Specific keywords and phrases commonly found in spam
- Patterns of email behavior, such as reporting rates or suspicious links
3. Training the Model
Using the collected data, machine learning models are trained to recognize spam. This is done through:
- Supervised learning methods, where the model learns from labeled examples of spam and non-spam emails.
- Unsupervised learning techniques that allow the model to detect anomalies or patterns without prior labels.
4. Implementation and Continuous Improvement
Once the model is trained, it is deployed to filter incoming emails. Continuous learning is critical, as the spam landscape is constantly evolving. By regularly updating the model with new data and retraining it, businesses can stay ahead of emerging threats.
Benefits of Machine Learning in Spam Filtering
The integration of spam filtering using machine learning brings numerous advantages for businesses:
Enhanced Accuracy
Machine learning models can achieve high levels of precision in identifying spam. By learning from both successful and unsuccessful filters, these systems continuously improve, reducing false positives and ensuring that legitimate emails are prioritized.
Scalability
As organizations grow, so too does their email communication. Spam filtering using machine learning can easily scale to accommodate increased email volume without compromising performance, making it ideal for businesses looking to expand.
Cost Savings
Implementing effective spam filtering can vastly reduce the costs associated with data breaches and employee downtime. Additionally, by automating spam management, IT teams can focus on high-value tasks that contribute to the organization's growth.
Improved User Experience
With intelligent spam filtering, employees experience less email clutter, making their communications more efficient. When users can trust that their email systems screen out spam effectively, they can direct their attention to more productive tasks.
Choosing the Right Spam Filtering Solution
When considering a machine learning spam filtering solution, businesses should evaluate the following:
1. Product Features
Look for solutions that offer customizable filters, integration with existing systems, and advanced security features.
2. Provider Reputation
Choose a vendor with a proven track record in email security and machine learning technologies. It’s essential to engage with reputable providers to ensure reliable protection.
3. User Feedback
Seek out user reviews or case studies showcasing the success of the spam filtering solution in real-world applications.
4. Support and Resources
A good vendor should provide ongoing support, resources for best practices, and clear documentation on how to optimize spam filtering settings.
Conclusion: Embrace the Future of Email Security
In a world where spam is not only an inconvenience but a legitimate threat to business security, spam filtering using machine learning is no longer optional—it is a necessity. As organizations strive to improve their IT services and security systems, the implementation of smart, adaptive filtering techniques can lead to significant improvements in efficiency, safety, and user satisfaction.
For businesses aiming to adopt machine learning solutions, it is imperative to partner with experts who understand the nuances of email management and are equipped with cutting-edge technologies. By choosing to invest in robust spam filtering, companies can not only safeguard their operations but also pave the way for thriving in the digital age.
Contact Us for More Information
If your organization is ready to enhance its spam protection mechanisms and improve overall security through spam filtering using machine learning, contact Spambrella today. Together, we can develop a personalized solution that meets your unique business needs, ensuring that your communications remain secure and efficient.